Federated data networks to boost collaboration across data silos
Sherpa.ai platform allows to build and train models collaboratively while maintaining data at the custodian firewall. Sherpa.ai provides enterprise-grade controls for custodians to remain in control of their data
Stop sharing data
In most real world scenarios data is decentralized across data silos (e.g. data located in users’ smartphones, hospitals, or banks) and can’t be shared which make collaboration between parties impossible. Sherpa.ai allows to build and train models collaboratively while preserving privacy. Models are trained locally at each node (e.g. at each hospital, at each bank or at each smartphone), and sharing only the model-updated local parameters and securely aggregating them to build a better global model (data never leaves the node and therefore is never shared).
Traditional Solution - Data is shared and centralized
Traditional Machine Learning approaches require to have all the training data in a single place. When data is distributed across silos, data needs to be shared with the data center before executing the training:
- When dealing with sensitive data, this is not compliant with regulation
- Once data is shared, control over it is lost
- Data is exposed in the communications and in the central repository
- Higher risk of breaching privacy, single point of failure
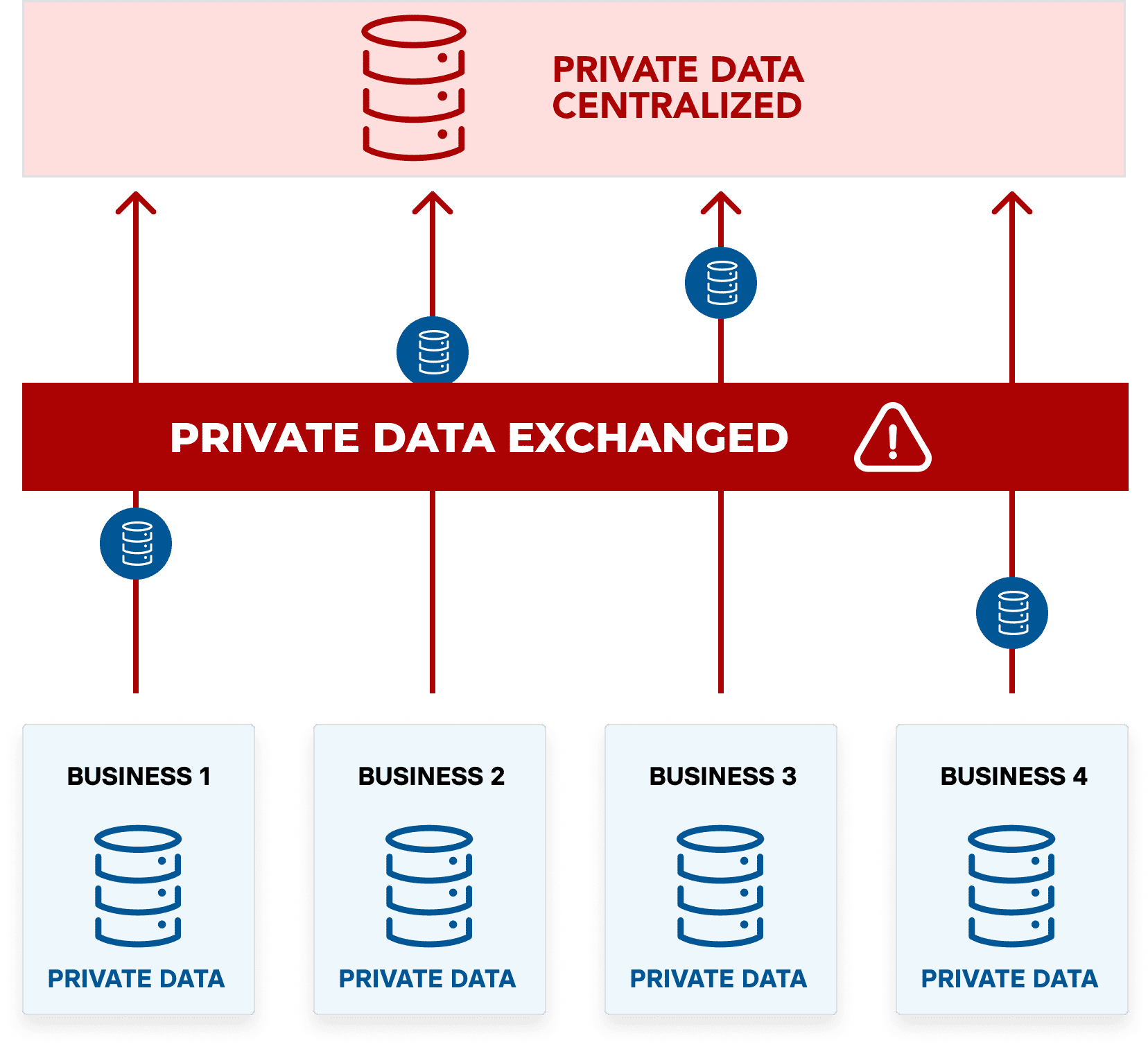
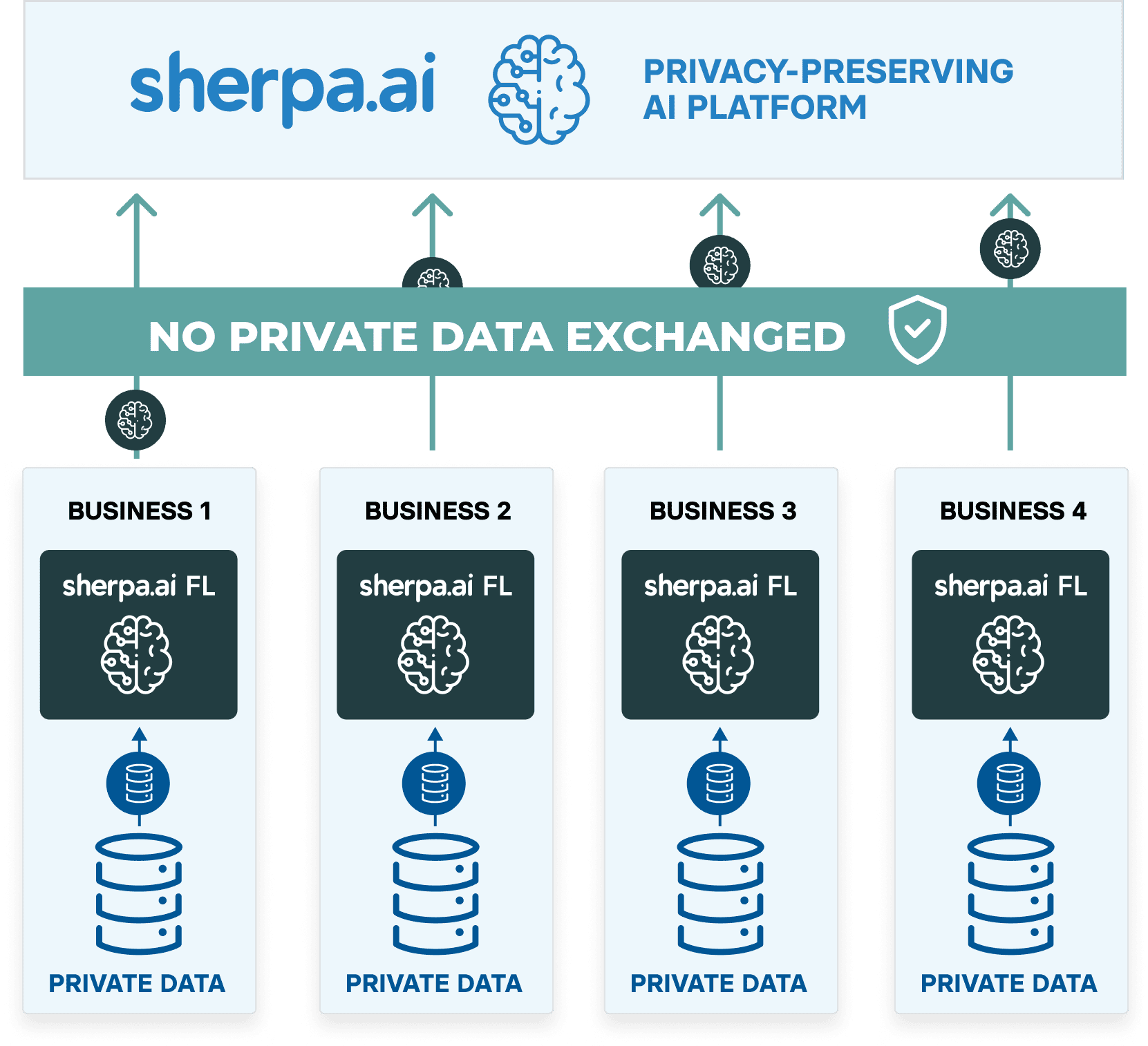
Sherpa.ai Solution - Data stays local
Sherpa.ai’s solution allows to run trainings without sharing data. The platform sends the models to the nodes to run local trainings. Then learinings are shared with the platform where they are aggregated to develop the global model.
- Unlocks the potential of data collaborations without sharing private data
- Privacy by design – no data is shared
- Regulatory-compliant – data never leaves the server of the parties involved
- Lower risk of data breaches. The attack surface is reduced

When does Federated Learning benefit Model Training
Our solution has transformative potential where data sharing limitations apply. Organizations encounter data sharing limitations from different reasons inclusing regulation, asset protection or data ethics among others

When data can’t be used or shared for regulatory reasons.
This is a common problem faced by large corporations whose data is stored in silos and can’t be shared due to regulation.
This is common in heavily regulated sectors like Financial Services or Healthcare.

When data contains confidential or sensitive information
Protected Health Information, financial records or any other identifiable information, that organizations are not willing to share

When different organizations want to take advantage of their data without sharing it.
For example, two competitive organizations could solve a common problem through collaborative model training, but they are not willing to share proprietary data with each other for competitive reasons. Federated Learning enables collaborative model training without sharing data.
Sherpa.ai benefits
Our solution can drastically improve results within weeks while ensuring the highest standars of privacy and security
Impact on results
Operational and financial impact from better AI models. Build and train models with heterogeneous data from diffrent parties.
Privacy & Security
Organizations must adapt to the new standards of privacy and regulations; regulatory compliance diminishes execution risk and costs.
Plug & Play
Seamless deployment process to accelerate time to value.
No additional infrastructure required.
Quotes from our team
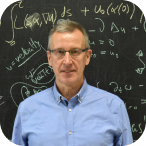
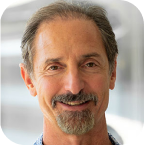